Fraunhofer Microelectronics
Energy-saving chips for artificial intelligence
The Fraunhofer Institutes EMFT, IIS and IPMS research energy-efficient neuromorphic hardware.
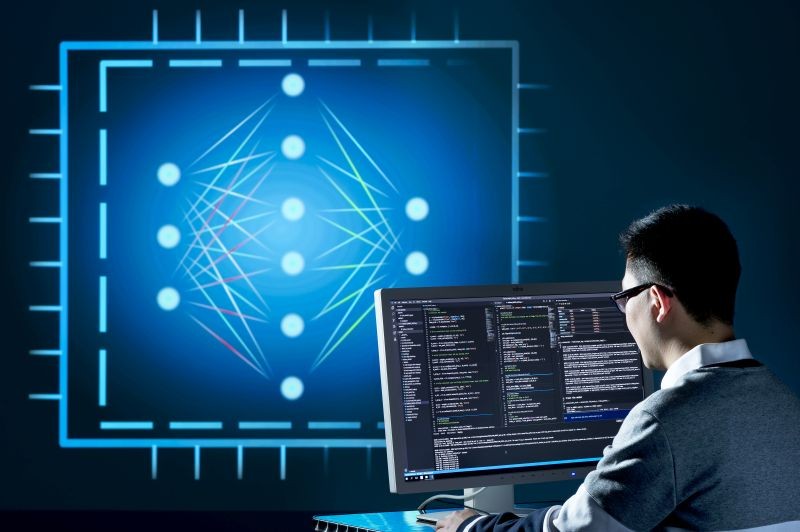
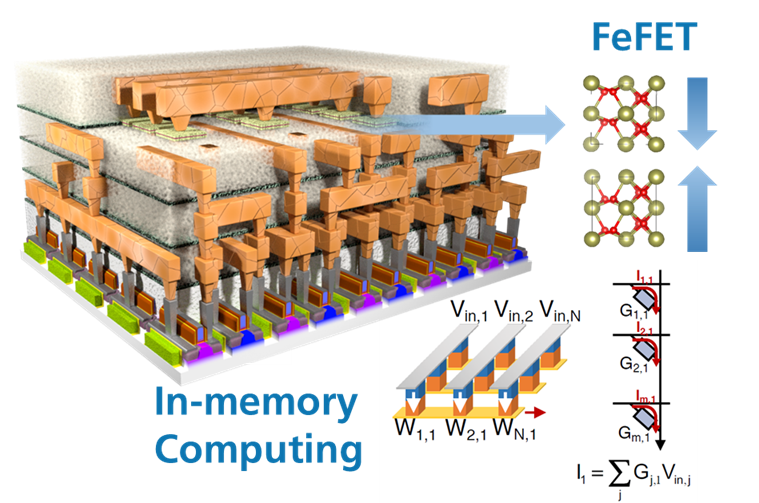
Neuromorphic hardware uses specialized computing architectures that reflect the structure (morphology) of neural networks from the bottom up: When we think, our brain processes a vast amount of information. This is made possible by billions of nerve cells interconnected by billions of synaptic connections. Neuromorphic computing – a key technology for artificial intelligence – imitates the morphology of the brain by means of processing units that emulate the behavior of neurons and synapses. This increases the energy efficiency for AI applications, because at present the complex thinking power of computers still consumes a lot of electrical energy.
Within the ECSEL project TEMPO, researchers are therefore developing novel and energy-efficient neuromorphic systems for semiconductor chips. The hardware architecture of the chips profits from embedded non-volatile storage and in-memory computing overcoming the limited data rate transfer between processor and external memory (von-Neumann bottleneck).
The researchers use new integrated storage technologies in innovative concepts for the realization of analog and digital neuromorphic circuits.
Fraunhofer expertise
Within the ECSEL project TEMPO (Technology & Hardware for Neuromorphic Computing), the Fraunhofer Research Institution for Microsystems and Solid State Technologies EMFT, the Fraunhofer Institute for Integrated Circuits IIS and the Fraunhofer Institute for Photonic Microsystems IPMS are working on the realization of neuromorphic hardware.
Fraunhofer EMFT focuses on the development of key IPs for analog and mixed-signal signal processing for neuromorphic structures. In detail, these are power-saving neuromorphic computer chips in the 2x nm technology node. Together with Fraunhofer IIS, Fraunhofer EMFT is the central contact regarding the development of key components for analog and mixed-signal neuromorphic hardware.
In this project, Fraunhofer IIS will lay the foundations for analog and digital deep learning reference accelerator components that will be used in future IP core products and subsequent industrial and funding projects. Based on these foundations, ASIC-based mixed-signal accelerators consisting of digital and analog components will be realized. Fraunhofer IIS develops for the digital inference accelerator a decompressor unit. Fraunhofer IIS is responsible for both the coordination and introducing the architectural design of a flexibly programmable digital deep learning accelerator together with videantis GmbH.
The Fraunhofer IPMS works on the development and evaluation of power-saving neuromorphic computing chips in the 22 nm FDSOI technology node. The developers use new integrated memory technologies based on ferroelectric hafnium dioxide in innovative concepts for the realization of analog and digital neuromorphic circuits. Fraunhofer IPMS focuses on the development of memory technologies and IPs for analog and mixed signal processing in the memory cells to enable efficient calculations.
Fields of application
The chips designed and manufactured in the project are to be used primarily for classification tasks in image recognition systems as well as for processing other sensor data. The possible fields of application include the automotive, space and health sectors. The chips can reduce the power consumption of signal processing of mobile and portable sensor systems by several orders of magnitude.
This minimizes the energy consumption for complex computing power and enables a novel computer architecture for artificial intelligence applications.
About TEMPO:
The project is funded by the EU and by the German Federal Ministry of Education and Research (BMBF). Further information on the project is available at:
Last modified: